Staff profile
Matthew Wiecek
Research Postgraduate (PhD)
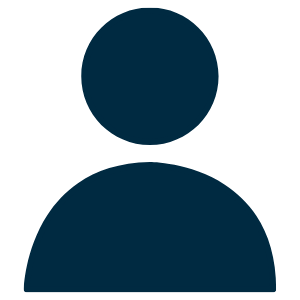
Affiliation |
---|
Research Postgraduate (PhD) in the Department of Geography |
Biography
My Research
For my PhD dissertation research, I am developing improved methods of using satellite images to monitor deforestation in South America, in collaboration with the Government of Guyana. For 12 years, Guyana has been working hard to minimize deforestation in its tropical rainforest. They get financial support from the Government of Norway, which is tied to the amount of deforestation that Guyana prevents. To measure deforestation, they have a web application that uses satellite images and machine learning to identify deforestation events with good but not perfect accuracy.
As a PhD student, I’m working in collaboration with Guyana to develop an improved method of automatically detecting deforestation events in real time. Deforestation is a major factor in environmental degradation, economic development, local quality of life and climate change, and my research will give national policymakers, local governments and climate change forecasters data that is more accurate, more precise and more detailed.
The policy context of my research is the REDD+ program. REDD+ stands for Reducing Emissions from Deforestation and Forest Degradation, and it is a United Nations-developed framework for developing agreements where a developed country provides financial support to a developing country in support of deforestation mitigation. A key part of a REDD+ program is a Monitoring, Verification and Reporting Systems (MRVS). An MRVS spells out how the developing country will measure deforestation, conduct audits and accuracy assessments, and report the amount of deforestation that they prevented or reversed in each year. The amount of funding provided is tied to the amount of deforestation that is prevented, hence the critical importance of accurate and detailed deforestation mapping.
The first REDD+ agreement was signed by Norway and Guyana in 2009. This program has grown considerably over the last 12 years. In the beginning, they only mapped change between forest and non-forest land cover types. Now, they map expansion and contraction of forested areas, changes in carbon stocks and biomass, detailed land cover/land use change mapping, collaboration with local communities, and more. As of 2016, they have also made scientific journal publications an objective of their REDD+ program.
The government agency responsible for the Guyana-Norway REDD+ agreement is the Guyana Forestry Commission. They have their in-house policymakers and technicians, and external contractors, who include Indufor Asia-Pacific and Prof. Danny Donoghue of Durham University. Danny has been a consultant in their accuracy assessment and auditing work for several years, and Indufor provides technical assistance in monitoring deforestation. To this end, Indufor has developed a web application that uses radar satellite images and machine learning to automatically identify deforestation events. Their accuracy and detail are good but have room for improvement.
Automatic identification of deforestation events will be done using image classification. Classifying images from different times, and looking at the difference, is how change is detected. In classification, the input is each pixel of an image, and the output is two values: forest, and non-forest. The goal is to set up a web application that takes a satellite image, and uses it to calculate where forest and non-forest are in the image.
There are two ways to do this. One way is to give the computer a model that tells it how to identify forest and non-forest in an image. This requires you to know what that model is in advance. The machine learning way is to give the computer the satellite image, and the correct answer, and have the computer figure out what the model is for you. If the computer did a good job and the model is accurate, then you will be able to give the computer a new image that it has not seen before, and be confident that the classification is accurate.
To be sure that the model is good, you need to test it. This is a two part process with five datasets:
1. Training. In this stage, the computer is given two datasets: the training data and the correct result. The computer fits a model to the data.
2. Testing. In this stage, there are three datasets: the testing data, the predictions, and the correct result.
1. The testing data is a different satellite image that the computer has not seen before. The testing image is given to the model fitted above, and the model makes predictions about which parts of the image are forest and non-forest.
2. The predictions are then compared with the correct result. The difference between the two is the accuracy of the predictions. If the difference is small, then the accuracy is high and the model is good. If the difference is great, then the accuracy is low and the model is bad.
The model is a machine learning classifier. Each classifier is a different mathematical/statistical process for classifying data, and different classifiers work well with different kinds of data. As a PhD student, I’m testing methods of combining different datasets and algorithms into one workflow that integrates their strengths and covers their weaknesses. My analysis will follow this flow:
• Testing single classifiers and datasets. Datasets include Planetscope, Sentinel-2, Sentinel-1 and others.
• Testing ensembles that combine different classifiers, in a way where one covers the weaknesses of another, and where their strengths can be combined.
• Testing ensembles that combine different datasets. Radar, visible light and infrared all record very different information, but this information is complementary, and a combined dataset with all of this information will see things not visible in the source datasets.
I’m working to provide something for three stakeholders:
1. I am working to produce original research that I can then submit as a dissertation, and get a PhD.
2. I am going to work to ensure that my published research meets the Guyana Forestry Commission’s objective of producing scientific journal publications as a result of the REDD+ program.
3. I am working to give Indufor recommendations on how to improve their process.
After this research is done, I’m interested in collaborating with other researchers who benefit from highly accurate and detailed forest change data. This includes climate change forecasting, forest ecology, the connection between deforestation and infectious disease outbreaks, and more.
Research interests
- Spatial data analysis using geographic information systems
- Remote sensing, especially sensor fusion, image classification, and time series analysis
- Environmental archaeology, especially human-climate interaction before the Industrial Revolution
- Reforestation and agroforestry science and policy, including land management practices of past cultures and present non-Western cultures
Publications
Conference Paper
- Improvements in arterial stiffness due to bean and pea consumption are determined by metabolic stateZahradka, P., Perera, D., Wilson, A., Wiecek, M., Bell, R., & Taylor, C. G. (2018). Improvements in arterial stiffness due to bean and pea consumption are determined by metabolic state. In Special Issue: Experimental Biology 2017 Meeting Abstracts. Federation of American Societies for Experimental Biology.
Journal Article
- Culture Contact and Diversity at a Site of the Northeastern Plains: An analysis of the ceramics and lithics at Snyder V (DhMg-6) in southwestern ManitobaWiecek, M., & Syms, E. L. (2020). Culture Contact and Diversity at a Site of the Northeastern Plains: An analysis of the ceramics and lithics at Snyder V (DhMg-6) in southwestern Manitoba. Manitoba Archaeological Journal, 30(1 & 2), 1-27.