Staff profile
Vicky Miles
Research Postgraduate - Computational Mechanics Node
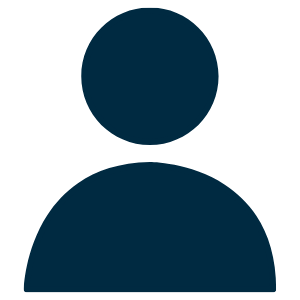
Affiliation |
---|
Research Postgraduate - Computational Mechanics Node in the Department of Engineering |
Biography
I am a PhD student in the engineering department, having started in 2020 after completing a MEng degree in electronic engineering. My research focuses on artificial intelligence, specifically in relation to the manufacturing industry.
Research Project
In recent years the manufacturing industry has been dramatically changing due to the widespread application of artificial intelligence techniques. Automated tools which can understand and interface with CAD models are of significant research interest due to the potential for improving efficiency in the design process, and so the manufacturing process as a whole.
This project involves the development of systems using recursive neural networks to automatically analyse STEP files (a standardised CAD model file format). Currently models are being trained to recognise specific features present in CAD models, with a longer-term goal of assessing general similarity of more complex models. This will lead to the development of tools to aid in 3D design, with a focus on aiding in standardisation of parts for manufacturing companies.
Publications
Conference Paper
Journal Article
- Miles, V., Giani, S., & Vogt, O. (2023). Approaching STEP file analysis as a language processing task: A robust and scale-invariant solution for machining feature recognition. Journal of Computational and Applied Mathematics, 427, Article 115166. https://doi.org/10.1016/j.cam.2023.115166
- Miles, V., Giani, S., & Vogt, O. (2023). Recursive Encoder Network for the Automatic Analysis of STEP Files. Journal of Intelligent Manufacturing, 34(1), 181-196. https://doi.org/10.1007/s10845-022-01998-x
- Miles, V., Gurr, F., & Giani, S. (2022). Camera-Based System for the Automatic Detection of Vehicle Axle Count and Speed Using Convolutional Neural Networks. International Journal of Intelligent Transportation Systems Research, 20(3), 778-792. https://doi.org/10.1007/s13177-022-00325-1